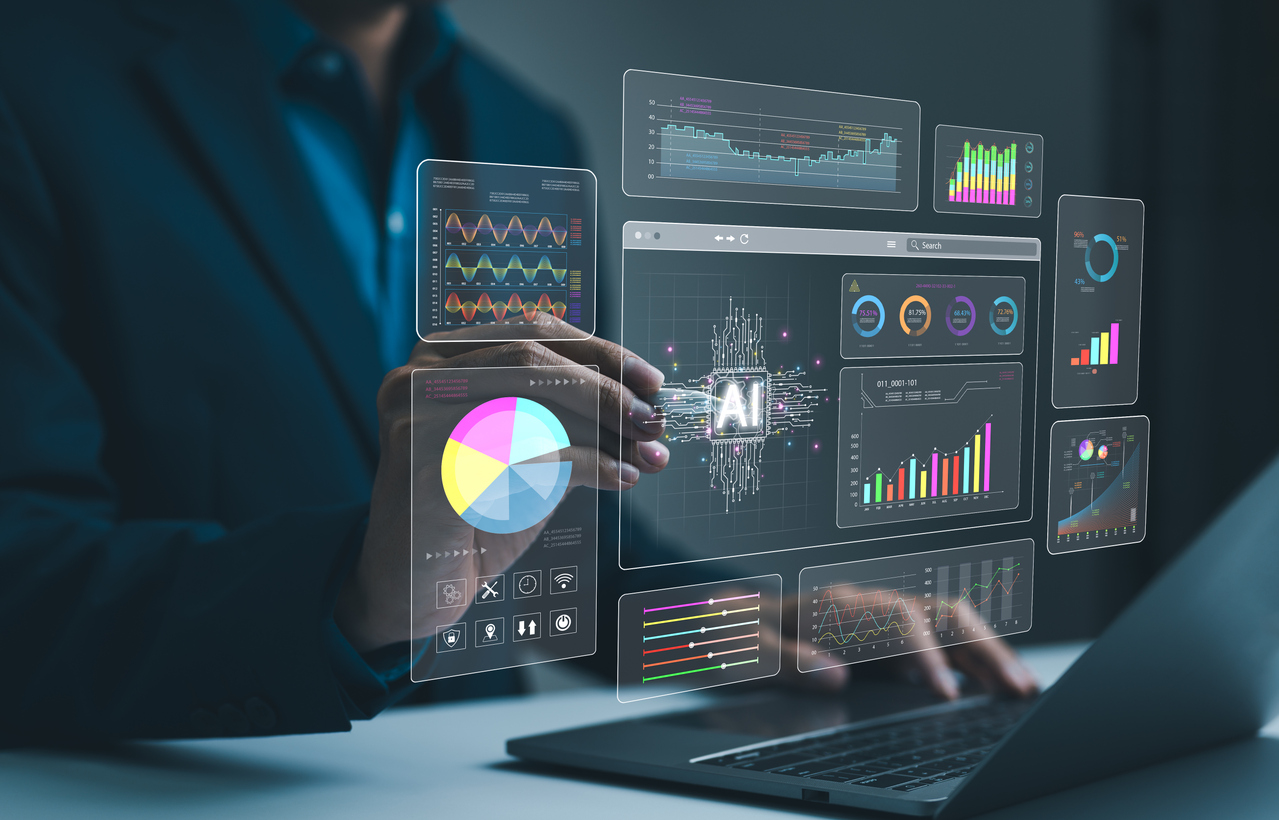
Modern organizations need advanced tools to deal with their expanding data collections because we now live in the big data era. Semantic data modeling tools represent key solutions because they manage the process of connecting meaningful data points between various points. Enterprise data management services combined with these tools allow businesses to achieve better insights while enhancing decision quality and foster innovation. This article investigates semantic data modeling tools and their relationship to enterprise data management services to achieve transformational outcomes.
The Definition of Semantic Data Modeling Tools Explains What They Represent
Semantic data modeling tools function as software applications that build organized data structures by representing how data elements relate to each other and their meaning, rather than merely storing and organizing data. Data modeling through semantic approaches differentiates itself from standard models by prioritizing context and understanding over storage designs and organizational structure. Such models help companies build intelligent data systems that enable better analysis opportunities.
Benefits of Semantic Data Modeling Tools
1. Improved Data Integration
The integration of data from multiple systems happens seamlessly through semantic modeling tools because they eliminate the existence of data silos to establish a comprehensive overview.
Organizations that implement enterprise data management services benefit greatly from this data consolidation method.
2. Enhanced Data Discovery
These tools enable users to understand data connections between points which raises their ability to find and retrieve necessary information.
This process allows better decision-making and it cuts down the time needed to find necessary data.
3. Contextual Understanding
These models supply a necessary context that enables people to understand what data means in context and its importance.
Such tools become essential for difficult datasets because they enable users to grasp relationships between data points needed to find valuable insights.
4. Scalability and Flexibility
Semantic data modeling tools have specific capabilities that enable them to work with big datasets while supporting the evolution of business requirements.
The system integrates perfectly with enterprise data management services to enable flexible data system growth and long-term protection.
5. Support for Advanced Analytics
By creating a structured and interconnected data framework, these tools enable advanced analytics, including machine learning and AI-driven insights.
This empowers businesses to uncover hidden patterns and trends.
How Semantic Data Modeling Tools Complement Enterprise Data Management Services
Enterprise data management services provide the foundation for effective data governance, storage, and security. When combined with semantic data modeling tools, these services become even more powerful. Here’s how:
- Unified Data Framework: Semantic modeling tools enhance the capabilities of data management practices by creating a cohesive and interconnected data framework.
- Improved Data Quality: By emphasizing relationships and context, semantic tools help identify and resolve data inconsistencies, improving overall data quality.
- Enhanced Collaboration: A shared understanding of data relationships fosters collaboration across teams and departments.
- Future-Ready Solutions: The combination of semantic modeling and enterprise data management services ensures that data systems are scalable, flexible, and ready for emerging technologies.
Key Features to Look for in Semantic Data Modeling Tools
When selecting a semantic data modeling tool, consider the following features:
- User-Friendly Interface: Tools should be intuitive and accessible to both technical and non-technical users.
- Integration Capabilities: Ensure the tool integrates seamlessly with your existing enterprise data management services and other data systems.
- Scalability: Choose a solution that can grow with your organization’s data needs.
- Support for Standards: Look for tools that support industry standards like RDF (Resource Description Framework) and OWL (Web Ontology Language).
Implementing Semantic Data Modeling in Your Organization
To successfully implement semantic data modeling, follow these steps:
- Assess Your Data Needs: Identify the areas where semantic modeling can add the most value.
- Choose the Right Tools: Partner with enterprise data management services providers to select tools that align with your goals.
- Train Your Team: Ensure your team understands how to use semantic modeling tools effectively.
- Monitor and Optimize: Continuously evaluate the impact of semantic modeling and refine your approach as needed.
Conclusion
Semantic data modeling tools are revolutionizing the way organizations manage and interpret their data. By creating meaningful connections between data points, these tools enhance the value of enterprise data management services, enabling businesses to unlock deeper insights and drive innovation. As data continues to grow in volume and complexity, the integration of semantic modeling and enterprise data management services will become essential for organizations aiming to stay competitive in a data-driven world. Embrace these tools today to transform your data into a strategic asset.